I have begun work on a new project, tentatively named GLOS, standing for Geographic Lens on Stories. The broad goal is to develop digital tools to aid in the comparative analysis of folkloric text traditions from around the world, with a focus on the relationship between stories and the places and societies they emerge from. One guiding premise is that the elements of folklore emerging from societies in a place are in some sense descriptive dimensions of that place. These elements include all categories of folkloric material, but the immediate concern of the GLOS project is stories: folktales, fairy tales, myths, epics, and so forth.
Qualifiers, more premises, and expectations
I do not have training in, or specialized knowledge of, folkloristics, nor of the anthropological themes closely associated with folklore. I approach this from a geographic perspective; I do have considerable knowledge and experience in representing place computationally, and I do have experience and skills in various natural language processing (NLP) methods. While I do expect GLOS work to elicit interesting distributional patterns, explanations for differences and similarities between story motifs and types will always include non-spatial factors I am not competent to analyze. Nevertheless, I expect to be able to generate and publish interesting patterns and will invite their analysis by others who do have interest and expertise.
Another premise of GLOS is that some of the emerging methodologies in NLP and machine learning, for example embeddings and large languages models (LLMs), can be usefully applied to folkloric text in novel ways.
Initial data preparation
I began by making digital text representations of the two canonical folktale indexes I'm aware of: Motif-index of folk-literature; (Stith Thompson, 1966) and The Types of International Folktales: A Classification and Bibliography (Hans-Jürg Uther, 2011). The latter is commonly referred to the "ATU index" because it folds together work from Antti Arne's The Types of the Folk-tale (1928), Thompson's motif index (TMI), and Uther's own considerable efforts. In each case, following OCR and cleaning, I parsed the raw, clean contents into structured and normalized formats amenable to storing in a relational database. The results include tables with the unique identifiers and text content of the TMI motifs and the ATU tale types.
There is also considerable metadata to be found in both the TMI and ATU, including references to the sources of cited tales, the societies and geographic areas they are associated with, and in the ATU, elaborate cross-referencing to related tale types and to the TMI motifs. These too have been parsed and stored in relational tables.
One of the outstanding challenges is establishing a geographic location for given motifs and tale types, so that they may be mapped and analyzed spatially. The data includes, variously 1) names of countries, provinces, historical regions; 2) names of indigenous tribes and societies; 3) languages. The task of establishing some kind of normalized gazetteer of place references is therefore quite complex and a comprehensive "where" attribute remains incomplete. For example, what is the geographic footprint of a tale type tagged only as "Hebrew" or "Spanish"? How can the locations of tribal territories or historical regions be represented given the dearth of spatial data for many of them? This is a major challenge that will require considerable research and expert consultation.
Initial computation
Leaving aside geography for the time being, I proceded to generate vector embeddings for the text of each of the 46,234 motifs in the TMI and 2,232 tale types in the ATU, using the OpenAI model, text-embedding-3-small. These are stored in relational tables, and wired to a very simple pilot web interface that allows a user to paste any piece of text into a form, and find the 10 motifs or tale types (a user choice) that are nearest neighbors in that 1536-dimension vector space.
Initial results
This first GLOS tool (tentatively Embedding Explorer) generates some interesting and essentially inadequate results that indicate other, more sophisticated approaches are necessary.
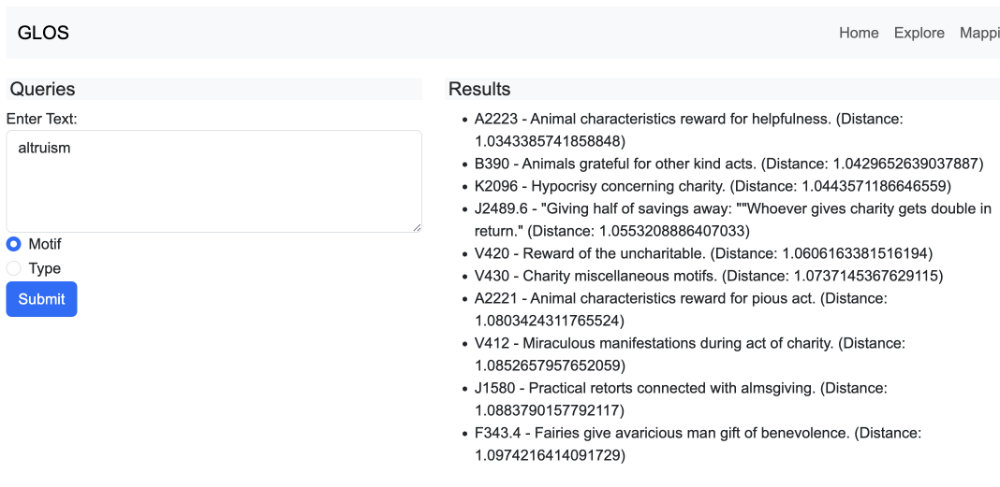
One goal of the tools is to find conceptual similarity without relying on term frequencies and co-occurence, as embedding technology promises. A search for a term like "altruism" should identify motifs and tale types that concern that concept in any of a number of societally differentiated ways.
For example, an altruistic act in one tradition might involve communal sharing of resources, while in another it might emphasize individual sacrifice for the greater good. Such nuances are important for understanding the cultural lenses through which altruism is interpreted. The tool succeeds to some extent - results for "altruism" include motifs and tales concerning charity, hospitality, and various acts of giving - but not all of the 10 nearest neighbors are recognizably conceptual neighbors, and it seems likely the method will miss some important matches.
Possible next steps
Some things I am considering:
-
Project corpora and fine-tuning. For instance, a corpus could include mythological texts from specific regions or curated examples of folk narratives with well-documented motifs and themes. Fine-tuning an LLM on this corpus could help improve the model’s ability to detect nuanced conceptual similarities and cultural context in motif analysis. This step might also involve aligning embeddings with external ontologies of folklore and mythology.
-
Enhance and extend the Embedding Explorer tool. Once results are improved, adding the ability to "drill down" and explore connections. The data includes relational information, like co-occurence of motifs and types, and could support various followup queries and graph navigation of those realtionships.
-
Once geographic information is made comprehensive, many interactive maps will be possible.
Feedback and suggestions are welcome!
The GLOS project has just begun, and I am feeling my way forward, reading disciplinary literature, coming up to speed on the fast-expanding AI methodologies that could help, and searching for prospective corpus material.
If any of this interests, or you have questions or comments (including critiques), please get in touch: karl [at] kgeographer [dot] org.